ENSPIRING.ai: Design a hybrid cloud infrastructure for and with AI
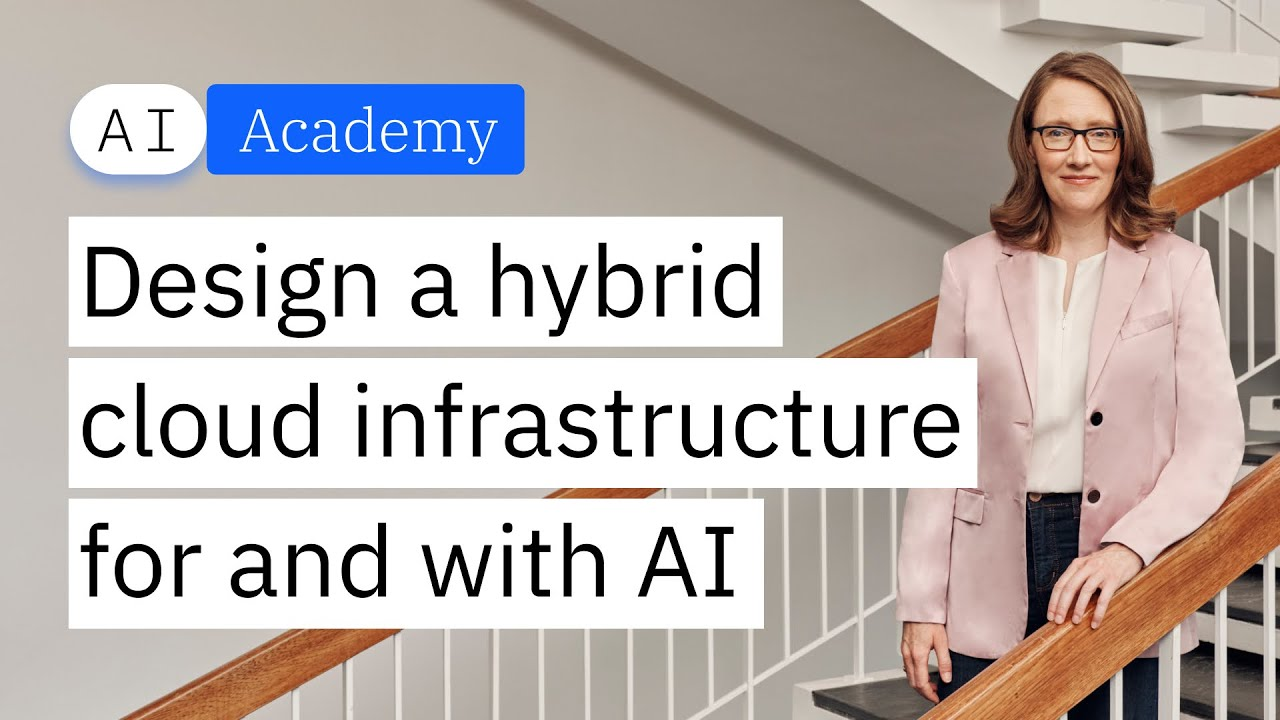
The video challenges the perception of "legacy technology" by highlighting how older systems can be an integral part of a hybrid cloud architecture, embodying the potential to enhance AI strategies. Hilary Hunter, CTO of IBM's infrastructure unit, explains that existing technology holds valuable data, vital for powering AI solutions. By integrating these tools as part of a well-designed hybrid cloud environment, businesses can effectively modernize their IT landscape and maximize AI potential.
Essentially, viewing older technology not as outdated but as legendary is crucial for AI's success. Companies can harness intentional hybrid cloud architectures to bring AI directly to where their data and applications are, thus optimizing data analysis both in-cloud and on-premises. This approach reduces data movement costs and enhances security, allowing for seamless data governance and integration.
Main takeaways from the video:
Please remember to turn on the CC button to view the subtitles.
Key Vocabularies and Common Phrases:
1. legacy technology [lɛɡəsi tɛkˈnɒlədʒi] - (noun) - Older software or technology that remains in use, often perceived as outdated but still functioning. - Synonyms: (outdated technology, old systems, traditional technology)
When you hear the term legacy technology, you might picture something old and outdated, like a crumbled roadmap that's stuffed into your car's console.
2. silos [ˈsaɪloʊz] - (noun) - Systems or departments that operate in isolation from others, often impeding data sharing and collaboration. - Synonyms: (isolation, separation, stand-alone)
That's why breaking down data silos and creating consistent environments across your IT estate are key to realizing the true potential of AI.
3. hybrid cloud [ˈhaɪbrɪd klaʊd] - (noun) - A computing environment that utilizes both private and public cloud services, enabling data and application integration. - Synonyms: (combined cloud, dual cloud, integrated cloud)
By choosing an intentional hybrid cloud architecture, you can bring your AI to wherever your data and applications already reside.
4. elasticity [iˌlæˈstɪsɪti] - (noun) - The ability of a system to scale resources up or down as needed, often dynamically. - Synonyms: (scalability, flexibility, adaptability)
The most important elements for system stability are elasticity, resiliency, and security.
5. resiliency [rɪˈzɪliənsi] - (noun) - The ability of a system to recover quickly and continue operating despite failures or disruptions. - Synonyms: (durability, robustness, toughness)
For resiliency, have high availability and disaster recovery in place.
6. devops [dɛːvəʊps] - (noun) - A set of practices combining software development and IT operations aimed at shortening the development lifecycle and providing continuous delivery with high software quality. - Synonyms: (agile operations, continuous deployment, dev and ops integration)
When we talk about modernizing your operations, we're talking about implementing platform engineering practices, formerly devops, devsecops, etcetera.
7. observability [əbzˌɜːˈveɪləˌbɪlɪti] - (noun) - The capacity of a system to provide insights into its operations through the analysis of logs, metrics, and traces. - Synonyms: (monitoring, tracking, detection)
Other crucial steps to modernizing your operations are to leverage infrastructure as code compliance as code, modern application observability, and automated workload.
8. governance [ˈɡʌvərnəns] - (noun) - The establishment of policies and continuous monitoring of their consistent implementation by the members of an organization. - Synonyms: (regulation, oversight, control)
With governance built in and integrated across this landscape from day one, your data can be organized and governed without moving it.
9. latency [ˈleɪtnsi] - (noun) - The delay before a transfer of data begins following an instruction for its transfer. - Synonyms: (delay, lag, wait time)
The result is substantially different latency improvements that can change business outcomes.
10. data cataloging [ˈdeɪtə ˈkætəlɒɡɪŋ] - (noun) - The process of creating an organized inventory of data assets, enabling easy data management and accessibility. - Synonyms: (data indexing, information cataloguing, data organizing)
The time is truly now to put in place robust data cataloging and data governance systems.
Design a hybrid cloud infrastructure for and with AI
When you hear the term legacy technology, you might picture something old and outdated, like a crumbled roadmap that's stuffed into your car's console. But in this episode, I'll explain how your existing technology, as part of a hybrid cloud by design architecture can be the engine that powers your AI strategy. Your existing technology holds your data, and your data is the basis for your AI.
Welcome to AI Academy. I'm Hilary Hunter, CTO of the IBM infrastructure unit and general manager of innovation. I work on the computers that you likely never see, and ideally shouldn't even know exist because they just work. Let's explore how to design a hybrid cloud architecture that works for and with your AI and your data. Often it leaders think about their legacy technology, for example, older applications, mainframe and uncoordinated instances of public cloud usage, as a hindrance to innovation. It can be viewed as being not agile, as a drain on budgets, and incapable of keeping up with the pace of change.
But AI doesn't exist in a vacuum. It gets its smarts from historical information combined with current and instantaneous data to work effectively. That's why breaking down data silos and creating consistent environments across your IT estate are key to realizing the true potential of AI. Let's go back to that map analogy that I started with. Imagine you're on a road trip trying to get from point a to point b in your journey. But imagine that there's big ink blots all over the map, removing critical information and making it impossible to decipher the roads that connect you to your destination. In this situation, you simply don't have all the information to be effective and efficient.
Alternately, if your systems aren't integrated, if your data isn't integrated, if legacy, it isn't modernized and brought to the table. In this AI conversation, that's like removing all of the interstates. You no longer have the superhighway to get you where you need to be. You're stuck on the back roads and you can easily lose your way because you're not able to utilize the best technologies and all of your data. You lose macro opportunities to solve big and bigger problems and get your business where it needs to go.
Simply put, don't think about your existing tech as legacy. Think about it as legendary, how each piece can help lead the AI journey to gold if it's used properly in this AI era, it's time to rethink your cloud strategies. By choosing an intentional hybrid cloud architecture, you can bring your AI to wherever your data and applications already reside. You'll have the ability to analyze the data in an optimized, organized way in the cloud and on premises. With governance built in and integrated across this landscape from day one, your data can be organized and governed without moving it, avoiding data movement costs and potential security compromises.
But if instead you don't leverage an intentional hybrid approach, then your data is constantly being moved to where the AI is, training to your desired outcomes. Tuning to your historical insights and applying AI to your current information takes time, money and work. Moving all of this scattered data can become arduous and expensive very fast, like a GPS trying to decipher the best route in a constantly changing underlying map. Implementing an intentional hybrid architecture lets you tackle AI with confidence, consistency, and enables you to course correct when needed.
The result is substantially different latency improvements that can change business outcomes, meaning being able to get on and use the superhighway cost efficiencies, meaning taking the most efficient route from point a to point b, and better insights. This is then far beyond just having a paper roadmap. This is providing your company with a GPS, a system which is adaptable and understands the third and fourth dimensions, like traffic and road conditions that don't even exist on a 2d map. Here are four considerations that come to my mind.
First, make sure your systems are stable and ready for AI. The most important elements for system stability are elasticity, resiliency and security. For elasticity, be sure your environments have appropriate capacity for expansion for resiliency, have high availability and disaster recovery in place. Because AI applications will become essential to your business. As you plan for AI to begin to operate on and leverage key data, this is an important time to check up on your cybersecurity management.
Next, adopt modern operations techniques. When we talk about modernizing your operations, we're talking about implementing platform engineering practices, formerly devops, devsecops, etcetera. Getting good at these will help the speed of AI creation and deployment. Other crucial steps to modernizing your operations are to leverage infrastructure as code compliance as code, modern application observability, and automated workload. Rebalancing these steps will help you spend less on IT management and give you more time to focus on building smarter applications.
Be sure to provide access for the data while many enterprises have been through multiple rounds of data management efforts in the past, the time is truly now to put in place robust data cataloging and data governance systems. These provide appropriate and seamless access for data. For creation of AI capabilities, API based modernization inserting points into an application where data and capabilities can be accessed is essential lastly, optimize end to end deployment. AI deployment is often feared to cause cost increases, but I want to put those fears to rest.
There are answers. We've seen that automated workload optimization can help take out 30% or more of GPU usage through management of system resources alone, and use of smaller, tailor fit AI models is key to cost management. Most importantly, having hybrid cloud solutions that enable you to choose where your AI runs means you can match up data with AI for a cost efficient, latency optimized solution.
What are the biggest roadblocks for technology leaders when it comes to AI? First, it can certainly take some imagination to see that you don't need to rewrite and rework everything that AI can be integrated into existing systems and workflows. But it's indeed very likely that your existing systems have powerful, secure and data rich capabilities that can give you a competitive edge. Today, AI can be deployed to great effect even within a mainframe hosted application. It can be deployed on premises, on systems hosting your critical data in the cloud, or at the edge.
When you power your enterprise with hybrid cloud capabilities, tailor fit models, governance and best practices, you can drive real value with AI. Implementing intentional hybrid cloud architecture is a business critical decision that ensures your systems are well protected, streamlined and optimized. Every new journey has its risk, but if you don't go, you'll never grow.
In IBM CEO Arvind Krishna's 2024 think keynote, he gave us a preview of a new class of generative AI assistance for advising and helping users of the mainframe, helping the IT operator to do their job better, and making the system smarter across the enterprise IT landscape. New AI assistance will range from helping coders to transform enterprise applications, to hybrid cloud assistance that facilitates system optimization, to code explainers that help developers understand and document applications through natural language. These are some of the many innovations and accelerations your company can take advantage of by adopting a hybrid cloud by design architecture for your AI.
And the time is now. Being able to get from point a to point b is the beginning of any road trip. So is making the most out of your existing IT resources to get the most from your AI, whether on well traveled routes or lands beyond your imagination. With the right tools and the ability to see the road ahead with clarity and confidence, it's gonna be a legendary journey. Ready? Let's go.
Technology, Innovation, Ai, Cloud Computing, Data Integration, Ibm, Ibm Technology
Comments ()