ENSPIRING.ai: Google DeepMind Executive on AlphaFolds Progress
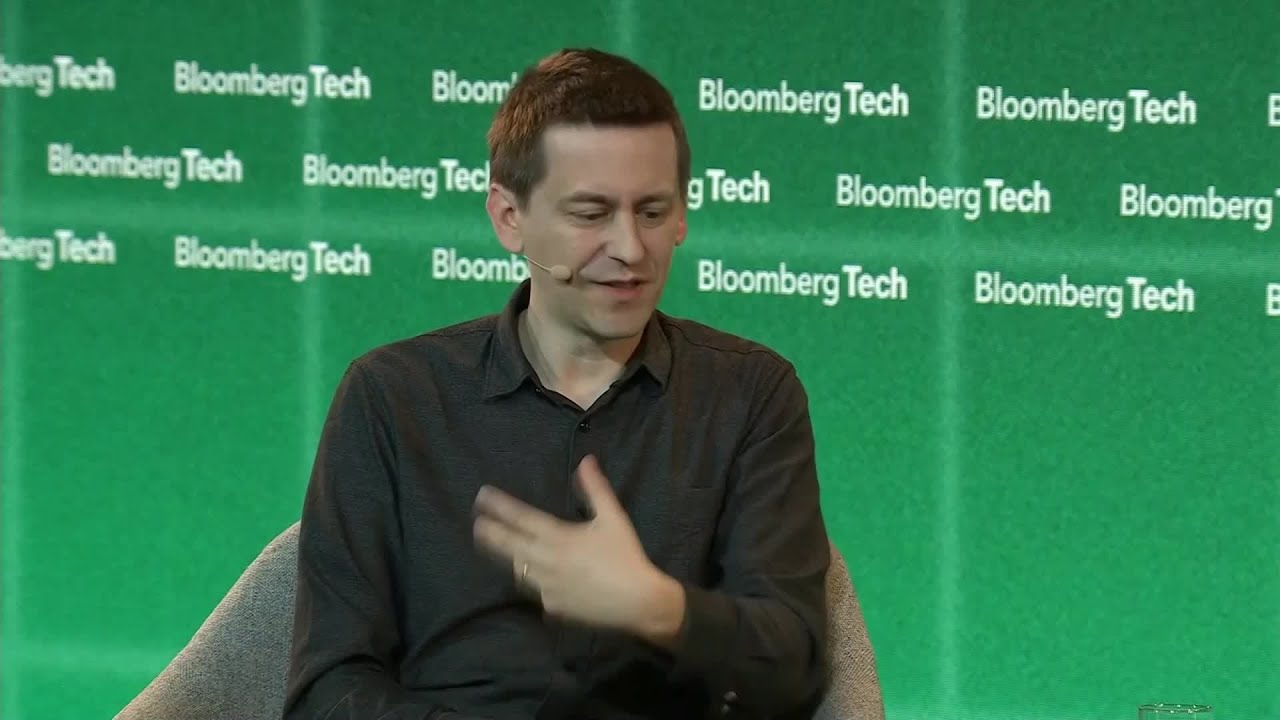
The video discusses the prestigious Nobel Prize awarded to John for his work on AlphaFold, a project that leverages AI to predict the 3D structures of proteins from amino acids. John's innovation, significant to the scientific community, exemplifies how AI is transforming fields like chemistry and physics. The excitement stems from AI's potential to offer rapid solutions to scientific queries, previously addressed through painstaking experiments. John's surprise at winning the Nobel Prize highlights the unpredictable nature of such recognition in the scientific community.
Viewers gain insight into AlphaFold’s significant contributions to structural biology and drug discovery. The tool is revolutionizing how scientists predict protein structures, significantly reducing the timeline and resources needed for experiments, thus facilitating faster hypothesis testing and advancements in drug delivery methods. Despite current limitations, such as the lengthy drug discovery timelines, AlphaFold's early-stage contributions are paving the way for accelerated progress in scientific research.
Main takeaways from the video:
Please remember to turn on the CC button to view the subtitles.
Key Vocabularies and Common Phrases:
1. co-awarded [ˌkoʊ əˈwɔrdɪd] - (verb) - To receive an award jointly or with another party. - Synonyms: (jointly awarded, shared, mutual recognition)
In case you haven't heard, John was co awarded the 2024 Nobel Prize in Chemistry for his work on alphafold.
2. statistical mechanics [stæˈtɪstɪkəl mɪˈkænɪks] - (noun) - A branch of physics that uses statistics to explain the behavior of systems composed of large numbers of particles. - Synonyms: (statistical physics, thermodynamics, probabilistic physics)
The Nobel Prize in Physics is a really wonderful prize that recognizes kind of scientists that studied how learning can arise from physical systems, how it's kind of within the laws of what's called statistical mechanics or physics.
3. x-ray crystallography [ˈɛks ˌreɪ ˌkrɪstəˈlɑgrəfi] - (noun) - A technique used for determining the atomic and molecular structure of a crystal by scattering X-ray beams at them. - Synonyms: (crystal analysis, X-ray diffraction, structural examination)
And the experiment that we predict is called, for example, X ray crystallography.
4. blueprint [ˈbluˌprɪnt] - (noun) - A detailed plan or outline that guides the construction or execution of something. - Synonyms: (scheme, plan, design)
If you hear that DNA is the blueprint of life, well, it's the blueprint basically for how to build proteins.
5. contractile injection system [kənˈtræktaɪl ɪnˈdʒɛkʃən ˈsɪstəm] - (noun) - A biological mechanism used by some organisms to inject substances into cells in a precise manner. - Synonyms: (injection apparatus, delivery mechanism, cellular syringe)
And they used Alphafold to look at the structure of this and find the piece that targets this, what's called contractile injection system.
6. nanoscopic [ˌnænoʊˈskɒpɪk] - (adjective) - Referring to dimensions on the nanometer scale, typically involving atoms and molecules. - Synonyms: (minuscule, atomic-scale, microscopic)
And I think, you know, some of the questions I'm very interested in are how do we go beyond this kind of narrow world of proteins and atoms and think just a little bit wider, Think about the cell, think about how proteins interact with each other, how we start to look at the cell and data around it, and how do we connect these predictions made at the kind of nanoscopic scale out to the microscopic scale?
7. incremental progress [ˌɪnkrəˈmɛntəl ˈprɑːɡrɛs] - (noun) - Advancements made through gradual steps rather than significant breakthroughs. - Synonyms: (gradual development, step-by-step improvement, iterative advancement)
But then you have to make incremental progress
8. calibrated [ˈkælɪˌbreɪtɪd] - (adjective) - Adjusted or marked so as to measure accurately. - Synonyms: (aligned, measured, tuned)
In fact, it gave very, what's called calibrated, but he gave a very clear answer.
9. emulating [ˈɛmjəˌleɪtɪŋ] - (verb) - To strive to equal or surpass, especially by imitating. - Synonyms: (imitating, mirroring, replicating)
And for some problems like emulating what humans can do, we're making progress, we're going out toward really general learning systems.
10. crossroads [ˈkrɔsˌroʊdz] - (noun) - A point at which a crucial decision must be made that will have far-reaching consequences. - Synonyms: (juncture, pivotal moment, intersection)
It's weird, I feel like crossroads of two moments.
Google DeepMind Executive on AlphaFold’s Progress
First of all, we got to start with congratulations on your amazing win. In case you haven't heard, John was co awarded the 2024 Nobel Prize in Chemistry for his work on alphafold. It uses AI to predict the 3D structure of a protein from its chain of amino acids. Yeah, let's give it up. Okay, so tell us about this win. What you just heard was a little bit of a call in which they told him that he won. And it was, it was funny because you go on to say you thought you had a 10% chance of winning. Tell us what you meant by that and also how you felt when you found out about this.
So, you know, for a long time, or I, a long time ago, when I was an undergrad or in grad school, I remember I had read this wonderful speech by Richard Hamming, who's an incredible computer scientist, you know, you and your research. And he says that you should, you know, scientists should try and do, you know, research that's worthy of a Nobel Prize. Right? Something that kind of really changes how we think about the science or really enables us to do new things. And, you know, the prize itself is only given to kind of one discovery per year. And. And you never really know. And there's so many incredible pieces of science that come out kind of. You know, we had known for some time that this mattered to scientists. We had really seen it. We had seen something like 30,000 papers that had cited our work and used it and really used it to do their science. Right? We build tools that enable other people to do science. But it was kind of hard to imagine, really, that we'd get this kind of call from Sweden. And I remember thinking kind of, you know, maybe 1 in 10, right? People talked about it. It was starting to be kind of discussed as something that could get a Nobel. But I thought not really. And in fact, I knew the call came about an hour before the press conference. And so it had gotten to 30 minutes before the press conference. And I remember I turned to my wife and I said, well, I guess not this year. And not 30 seconds later had this phone call with the Swedish area code. And I was really glad it wasn't the meanest prank ever. And just absolutely kind of unbelievable and extraordinary that they chose to recognize us this year and such a prestigious prize and recognize what AI is doing for scientists.
So some people were surprised to hear that a Nobel Prize for Chemistry and also one for physics was awarded for work that's very focused on AI. What do you think it indicates? Do we need a New category for Nobel Prizes. Does it show how much AI has been integrated into all kinds of research? I think, and there's really the two different Nobel Prizes are for two different aspects. The Nobel Prize in Physics is a really wonderful prize that recognizes kind of scientists that studied how learning can arise from physical systems, how it's kind of within the laws of what's called statistical mechanics or physics. I used to be a physicist. I'll try not to be too technical, but. But really like, how does learning arise? And kind of the early moments, and I remember a lot of that work was actually very influential to me when I was a younger scientist. And then the Nobel Prize in Chemistry is about kind of computational science being able to solve problems we need to solve. And it's really about how AI. And really the program we worked on, AlphaFold, is solving problems that we don't otherwise know how to solve. And I think that it's about that. It matters to scientists who don't necessarily have an interest in AI or in tech, but they have questions about how does this piece of the body work? And then they turn to our tools to get answers to those questions. And so it's really about the meaning of this for scientists.
Let's talk about AlphaFold in progress. What kind of progress have we seen with AlphaFold thus far? So what we do, this problem that we work on, so we really predict the results of a scientific experiment. So we're trying to use a computer to say this is what you're likely to get if you were to do this experiment. And the experiment that we predict is called, for example, X ray crystallography. It's taking pictures of proteins, and proteins are these nanomachines of your cell. If you hear that DNA is the blueprint of life, well, it's the blueprint basically for how to build proteins. And these are a few thousand atoms big, smaller than the wavelength of light. And when they're made, they're made as basically a string that folds up itself just due to the laws of physics, into this intricate 3D shape. And that's what functions. For example, there are proteins in your muscle cells, and when your muscles contract, it's basically pulling via these proteins. And so scientists have long tried to get the shape of these proteins and understand it just from what you can read out of the DNA. And it still takes scientists a year or more to get a single answer right. It's an incredibly difficult experiment, if you want to put it in economic terms. It's about $100,000 in investment on Average to get one of these structures. And but most importantly, it's often gating. Scientists want to study Alzheimer's or they want to study, you know, there's a lovely example on fertilization or many others. They want to study these processes and then they have to take a year to do this experiment. And we've built an AI system that gives very accurate predictions as well as a strong indication of how confident we are in those predictions, and does it in about five or 10 minutes. So it's really taking a year to five minutes.
So it's shortening that timeline. But then what happens after that? Are we seeing any meaningful drug discovery as of yet, or anything else that AlphaFold has led to that you can point to? So we're seeing some really wonderful applications across both structural biology and drug discovery. And what we really see is that scientists are using this tool to discover new hypotheses that they then go test in the lab. One really wonderful example, I guess, is from Feng Zheng's lab at, I believe, mit. And they were studying this particular system found in possibly bacteria, I can't remember which injects one protein into a cell in a very targeted manner. And they used Alphafold to look at the structure of this and find the piece that targets this, what's called contractile injection system, and they swap it out for a new design. And they showed that in mice, they can inject a particular protein into a specific type of cell in the mouse brain. So they're developing new types of drug delivery systems as the ultimate goal. We're seeing also with the latest versions of AlphaFold, that we can predict how drugs will attach to proteins. And people are starting to say, okay, well, now I can do rational design of drugs more quickly. And we're seeing really, I think, kind of still at the early stages, drug design still takes something like 10 years. But we're seeing a lot in which people are integrating these predictions and skipping experimental steps to get further along drug development.
When you think about the future, what kind of timetable do you think there might be for getting, let's say, some drugs, A drug on the market that would be used initially, AlphaFold would be used initially to help produce that. So I think we're already seeing the influence on early stage drug design. Now, of course, you still have to do clinical trials. There's nothing that skips clinical trials. We need to do this. So you're still, I think, seeing, you know, five, seven plus years to get the first drugs to market. But what I think you'll also See is really an acceleration and we'll see this, we'll see many other tools. I also think AlphaFold is probably a first tool, but we'll have many others that together will help us compress early stage drug discovery quite dramatically. But I think really the first we'll see is probably five, seven years just because of clinical trial timelines.
Okay. In a recent essay that he published, Dario Amade of Anthropic praised Alphafold and wrote about a future in which you use AI to perform, direct and improve upon nearly everything biologists do, which is, which is quite broad. And that the use of AI tools such as AlphaFold and others could shrink the next 50 to 100 years of biological progress into 5 to 10 years. That seems like a lot of shrinking. How likely does that sound to you? I think it, some parts of it I think are quite reasonable. I think the time frame in which you'll start to see that happen, I'm not sure it will be within five years. It will depend, of course, on progress in AI. I think what we will get definitely, almost certainly is very powerful tools in specific areas. And we've actually already seen this in biology where we have, you know, very powerful tools to read DNA and then we build very clever experiments which end in reading DNA because we're so very good at it. So I think we'll see quite a lot of that and then I think it'll depend really on how much further do we get in general reasoning systems. And it is a very interesting point that AlphaFold is really showing that for certain kind of narrow problems in science, just like we've seen previously in say, narrow problems in games, AI systems can be vastly, vastly more effective than anything else we know how to build and than humans at the same task. Right. There's no humans that are good at predicting the structure of proteins. So I think if we are, you know, fortunate, we'll really see this acceleration within AI. And I won't, I can't guess 100 years in the future, but I believe it will be dramatic.
You mentioned with AlphaFold, it's working on something that people could do, but it's extremely time consuming, it's very expensive, it takes a really long time. It's not something that one person alone could do or could do very quickly. A lot of the AI systems that we see now are aimed at automating things that humans can do in a relatively short time span. A lot of task related work. Do you feel like we should be, are we maybe concentrating too much on that sort of short term work, should we be concentrating more on these longer term bets? I think there's a healthy mix. I think it is really the case that some of the places where we're going to see maybe some of the most dramatic within a single task improvements are probably going to be more narrow and scientific. We've really got this incredible general, incredibly general new technology that lets us learn from data. And for some problems like emulating what humans can do, we're making progress, we're going out toward really general learning systems. But we're already seeing the dividends more narrowly and I think that's going to be very exciting. And we also will benefit from all of the investment into GPUs and TPUs, into ideas and algorithms for how to learn. Those are all going to pay off enormously into scientific domains and other domains. And I think we'll see some really exciting, exciting work that isn't just making us faster at human tasks, but otherwise. And I think it's hard to say we're underinvested in anything in AI, but I think it will be a very exciting time.
So when you were doing the work on Alphold, it was, I mean at this point it was a number of years ago. So how has the experience of working on bleeding edge AI at Google changed over the past five years? I mean a lot has changed in AI. Do you feel like a team like yours could do work such as this that you could do five years ago? Could you do this work now? I think so. I think it's, you know, in a lot of ways gotten easier in part because it's been proved out like the notion that AI is really and within DeepMind and now Google. DeepMind. Of course, you know, Davis always believed in, always believed in the applications of science, but I think this notion that it will be absolutely transformative in fields has now been well established. In that way it's easier also, you know, compute has increased. There are lots of, lots of things that have actually improved. If anything has gotten harder, it's that there are so many people now working in it that you have to find the right niche. Although even I think still DeepMind has this advantage. Or at least we've always worked with really great teams. We've always been really focused and I think one of the just really exciting things as a person working in AI is how much influence a small group of people can have working on the right problem in the right way, that it's actually very small teams that build a lot of really great things.
Yeah, you Mentioned when we spoke the other day that it was quite a small team behind AlphaFold initially. How many people was it? It was about 15 that worked on it full time. Okay, so some, you know, support from the wider Google DeepMind. Org, but the core team is around 15. And in fact not even very few of us with much biology experience. I was, I guess biophysics for my PhD, but, you know, maybe the worst biologists in my group, but the second best on the AlphaFold team. So I think it really is a small group of people that can find the right problem and change the direction.
You mentioned Richard Hamming, you and your research. You mentioned it here. You also mentioned it in the call when they told you that you had won. And the idea that some people who win Nobel Prizes can then feel like they have to focus on big problems to solve at the expense of not nurturing smaller things that can turn into big things. So how are you thinking about avoiding that? What is one of those small things that you're working on that you hope will turn into something big? So I won't go too much into. I do try and find the right problems. I think four for me, you know, I have. It's weird, I feel like crossroads of two moments. One is kind of, you know, as you mentioned in Dario's essay, this notion that AI really, really transformed the sciences. And I do believe that. So then it's, oh, we should work on grand things because the sciences are about to be transformed. But then you have to make incremental progress. And I think one of the lessons of AlphaFold for me is, you know, we had this huge breakthrough, but on, say, our leaderboard, we moved 1% at a time and no idea was worth more than, you know, one point on this hundred point scale. And so, you know, by inches we got to where we are. And I think, you know, some of the questions I'm very interested in are how do we go beyond this kind of narrow world of proteins and atoms and think just a little bit wider, Think about the cell, think about how proteins interact with each other, how we start to look at the cell and data around it, and how do we connect these predictions made at the kind of nanoscopic scale out to the microscopic scale? But we'll see. And one of the things, you know, the other reason I don't like to talk about what I'm going to do next is I want the freedom to change it. And I think it's more important, especially as fast as technology is, to be responsive to the right thing to do. Tomorrow, which might not have been the right thing to do yesterday.
I'm going to start saying that to my editor. I don't want to tell you what I'm working on next because I want the free freedom to change it. Yeah, yeah. Well, once you say, everyone, I'm going to do this, then they'll ask you in a year or so, do we have Exactly. Yeah. Where is it? What's the timeline? Trust is one of the themes of this conference. I feel like it comes up more and more and more as consumers are increasingly accustomed to using AI in their daily lives. I mean, they were using machine learning in many ways previously, but it wasn't super, super obvious, I think, to a lot of people, except for until a few years from now. What have you learned from your work that we can apply more generally as far as helping people know when they can trust information that is generated with AI. So this was one of the things we worried a lot about as we were planning to release AlphaFold. And we were thinking like, how do we do this responsibly? And so, you know, and the thing that worried me is I worked in a mixed wet and dry lab with experimentalists and computationalists in my PhD and I didn't want someone to look at our prediction and go do the wrong thing for the next six months. And so what? And as we were coming out with this, we said, we need some way of knowing if it's right. And we actually ended up with a very kind of direct method, surprisingly direct, as we teach the algorithm to produce an answer and a prediction of what the error would be if you knew the right answer, of the answer it gave. Kind of like if you take a test and someone asks you, what grade do you think you got? And that worked extremely well. In fact, it gave very, what's called calibrated, but he gave a very clear answer. So it'll say, you know, I have to give some answer. But this part I believe, this part I don't believe. I maybe don't believe how these come together. And I've seen it really integrated into the work of scientists. And, you know, scientists are used to dealing with uncertain information. Scientists don't even necessarily trust experiments at other labs. And they've done, I think, a really good job at integrating the confidence that these AI systems produce. And it's an advantage that we have over large language models at the moment and integrating it into their practice in a very effective way. And I think it's going to be essential for scientific AI systems.
Do you think maybe we could use a little bit more of that in consumer oriented systems. Like if you get an answer from a chatbot, perhaps it should include a confidence score. I hope people will develop things like this. It becomes more complex if we can't. One of the nice things about science is that you can agree on the right answer at least after the fact. Right. You know, the measurement was performed. We resolved disputes by actually measuring. But I think it is something that is going to have to be integrated. We're going to have to kind of acknowledge that sometimes it's going to be confident, sometimes it's not going to be confident, much like sources on the Internet that we already do and we have to figure out how to come up with this kind of view of how sure a machine is and the answer. Thank you so, so much. It was really wonderful to talk to you and congratulations again. Thank you.
Artificial Intelligence, Chemistry, Nobel Prize, Alphafold, Scientific Research, Drug Discovery, Bloomberg Live
Comments ()